SCI Publications
2012
B.A. Zielinski, J.S. Anderson, A.L. Froehlich, M.B.D. Prigge, J.A. Nielsen, J.R. Cooperrider, A.N. Cariello, P.T. Fletcher, A.L. Alexander, Nicholas Lange, Erin D Bigler, Janet E Lainhart.
scMRI Reveals Large-Scale Brain Network Abnormalities in Autism, In PloS one, Vol. 7, No. 11, pp. e49172. 2012.
DOI: 10.1371/journal.pone.0049172
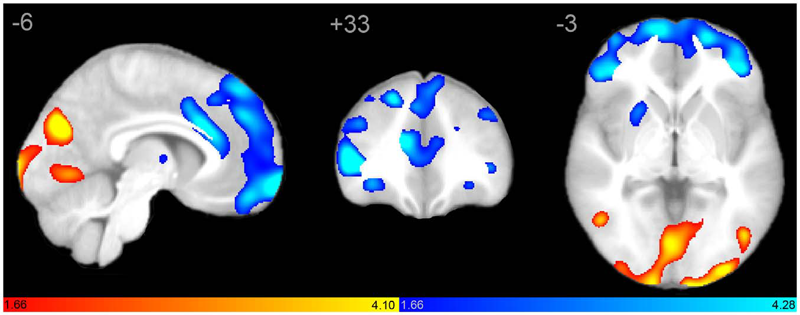
2011
J.S. Anderson, J.A. Nielsen, A.L. Froehlich, M.B. DuBray, T.J. Druzgal, A.N. Cariello, J.R. Cooperrider, B.A. Zielinski, C. Ravichandran, P.T. Fletcher, A.L. Alexander, E.D. Bigler, N. Lange, J.E. Lainhart.
Functional connectivity magnetic resonance imaging classification of autism, In Brain, pp. (published online). 2011.
DOI: 10.1093/brain/awr263
PubMed ID: 22006979
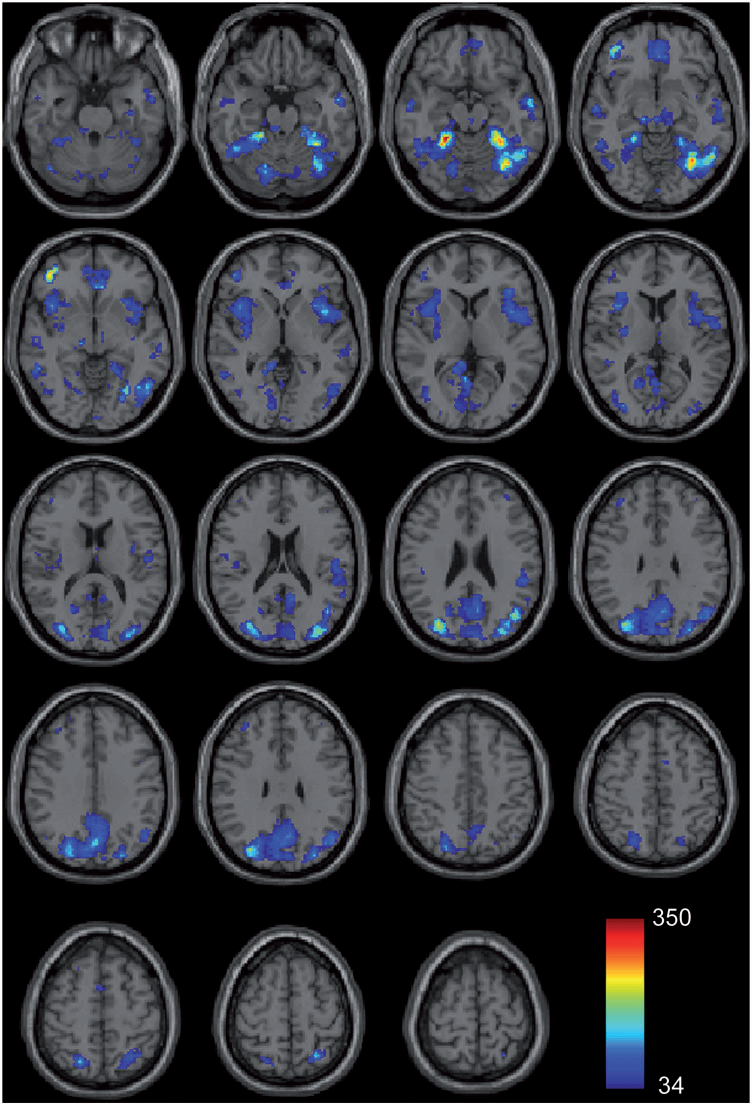
Group differences in resting state functional magnetic resonance imaging connectivity between individuals with autism and typically developing controls have been widely replicated for a small number of discrete brain regions, yet the whole-brain distribution of connectivity abnormalities in autism is not well characterized. It is also unclear whether functional connectivity is sufficiently robust to be used as a diagnostic or prognostic metric in individual patients with autism. We obtained pairwise functional connectivity measurements from a lattice of 7266 regions of interest covering the entire grey matter (26.4 million connections) in a well-characterized set of 40 male adolescents and young adults with autism and 40 age-, sex- and IQ-matched typically developing subjects. A single resting state blood oxygen level-dependent scan of 8 min was used for the classification in each subject. A leave-one-out classifier successfully distinguished autism from control subjects with 83% sensitivity and 75\% specificity for a total accuracy of 79\% (P = 1.1 x 10-7). In subjects less than 20 years of age, the classifier performed at 89\% accuracy (P = 5.4 x 10-7). In a replication dataset consisting of 21 individuals from six families with both affected and unaffected siblings, the classifier performed at 71\% accuracy (91\% accuracy for subjects less than 20 years of age). Classification scores in subjects with autism were significantly correlated with the Social Responsiveness Scale (P = 0.05), verbal IQ (P = 0.02) and the Autism Diagnostic Observation Schedule-Generic's combined social and communication subscores (P = 0.05). An analysis of informative connections demonstrated that region of interest pairs with strongest correlation values were most abnormal in autism. Negatively correlated region of interest pairs showed higher correlation in autism (less anticorrelation), possibly representing weaker inhibitory connections, particularly for long connections (Euclidean distance greater than 10 cm). Brain regions showing greatest differences included regions of the default mode network, superior parietal lobule, fusiform gyrus and anterior insula. Overall, classification accuracy was better for younger subjects, with differences between autism and control subjects diminishing after 19 years of age. Classification scores of unaffected siblings of individuals with autism were more similar to those of the control subjects than to those of the subjects with autism. These findings indicate feasibility of a functional connectivity magnetic resonance imaging diagnostic assay for autism.
P.T. Fletcher.
Geodesic Regression on Riemannian Manifolds, In Proceedings of the Third MICCIA International Workshop on Mathematical Foundations of Computational Anatomy (MFCA), Toronto, Canada, pp. 75--86. 2011.
P.T. Fletcher, J. Moeller, J. Phillips, S. Venkatasubramanian.
Horoball hulls and extents in positive definite space, In Algorithms and Data Structures, Lecture Notes in Computer Science (LNCS), Vol. 6844/2011, pp. 386--398. 2011.
DOI: 10.1007/978-3-642-22300-6_33
X. Hao, R.T. Whitaker, P.T. Fletcher.
Adaptive Riemannian Metrics for Improved Geodesic Tracking of White Matter, In Information Processing in Medical Imaging (IPMI), Lecture Notes in Computer Science (LNCS), Vol. 6801/2011, pp. 13--24. 2011.
DOI: 10.1007/978-3-642-22092-0_2
W. Liu, S. Awate, J. Anderson, D. Yurgelun-Todd, P.T. Fletcher.
Monte Carlo expectation maximization with hidden Markov models to detect functional networks in resting-state fMRI, In Machine Learning in Medical Imaging, Lecture Notes in Computer Science (LNCS), Vol. 7009/2011, pp. 59--66. 2011.
DOI: 10.1007/978-3-642-24319-6_8
N. Sadeghi, M.W. Prastawa, P.T. Fletcher, J.H. Gilmore, W. Lin, G. Gerig.
Statistical Growth Modeling of Longitudinal DT-MRI for Regional Characterization of Early Brain Development, In Proceedings of the Medical Image Computing and Computer Assisted Intervention (MICCAI) 2011 Workshop on Image Analysis of Human Brain Development, pp. 1507--1510. 2011.
DOI: 10.1109/ISBI.2012.6235858
A population growth model that represents the growth trajectories of individual subjects is critical to study and understand neurodevelopment. This paper presents a framework for jointly estimating and modeling individual and population growth trajectories, and determining significant regional differences in growth pattern characteristics applied to longitudinal neuroimaging data. We use non-linear mixed effect modeling where temporal change is modeled by the Gompertz function. The Gompertz function uses intuitive parameters related to delay, rate of change, and expected asymptotic value; all descriptive measures which can answer clinical questions related to growth. Our proposed framework combines nonlinear modeling of individual trajectories, population analysis, and testing for regional differences. We apply this framework to the study of early maturation in white matter regions as measured with diffusion tensor imaging (DTI). Regional differences between anatomical regions of interest that are known to mature differently are analyzed and quantified. Experiments with image data from a large ongoing clinical study show that our framework provides descriptive, quantitative information on growth trajectories that can be directly interpreted by clinicians. To our knowledge, this is the first longitudinal analysis of growth functions to explain the trajectory of early brain maturation as it is represented in DTI.
Keywords: namic
2010
E.B. Dam, P.T. Fletcher, S.M. Pizer.
Automatic shape model building based on principal geodesic analysis bootstrapping, In Medical Image Analysis, Vol. 12, No. 2, Note: Epub Feb 2 2010, pp. 136--151. 2010.
PubMed ID: 18178124
B.C. Davis, P.T. Fletcher, E. Bullitt, S. Joshi.
Population Shape Regression from Random Design Data, In International Journal of Computer Vision, Vol. 90, No. 1, Note: Marr Prize Special Issue, pp. 255--266. October, 2010.
DOI: 10.1109/ICCV.2007.4408977
P.T. Fletcher, R.T. Whitaker, R. Tao, M.B. DuBray, A. Froehlich, C. Ravichandran, A.L. Alexander, E.D. Bigler, N. Lange, J.E. Lainhart.
Microstructural connectivity of the arcuate fasciculus in adolescents with high-functioning autism, In NeuroImage, Vol. 51, No. 3, Note: Epub Feb 2 2010, pp. 1117--1125. July, 2010.
PubMed ID: 20132894
S. Gerber, T. Tasdizen, P.T. Fletcher, S. Joshi, R.T. Whitaker, the Alzheimers Disease Neuroimaging Initiative (ADNI).
Manifold modeling for brain population analysis, In Medical Image Analysis, Special Issue on the 12th International Conference on Medical Image Computing and Computer Assisted Intervention (MICCAI) 2009, Vol. 14, No. 5, Note: Awarded MICCAI 2010, Best of the Journal Issue Award, pp. 643--653. 2010.
ISSN: 1361-8415
DOI: 10.1016/j.media.2010.05.008
PubMed ID: 20579930
N. Lange, M.B. Dubray, J.E. Lee, M.P. Froimowitz, A. Froehlich, N. Adluru, B. Wright, C. Ravichandran, P.T. Fletcher, E.D. Bigler, A.L. Alexander, J.E. Lainhart.
Atypical diffusion tensor hemispheric asymmetry in autism, In Autism Research, Vol. 3, No. 6, pp. 350--358. 2010.
DOI: 10.1002/aur.162
PubMed ID: 21182212
W. Liu, P. Zhu, J.S. Anderson, D. Yurgelun-Todd, P.T. Fletcher.
Spatial Regularization of Functional Connectivity Using High-Dimensional Markov Random Fields, In Medical Image Computing and Computer-Assisted Intervention (MICCAI 2010), Vol. 14, pp. 363--370. 2010.
PubMed ID: 20879336
N.P. Singh, P.T. Fletcher, J.S. Preston, L. Ha, R. King, J.S. Marron, M. Wiener, S. Joshi.
Multivariate Statistical Analysis of Deformation Momenta Relating Anatomical Shape to Neuropsychological Measures, In Medical Image Computing and Computer-Assisted Intervention – MICCAI 2010, Lecture Notes in Computer Science (LCNS), Vol. 6363/2010, pp. 529-537. 2010.
DOI: 10.1007/978-3-642-15711-0_66
PubMed ID: 20879441
2009
M. Datar, J. Cates, P.T. Fletcher, S. Gouttard, G. Gerig, R.T. Whitaker.
Particle Based Shape Regression of Open Surfaces with Applications to Developmental Neuroimaging, In Medical Image Computing and Computer-Assisted Intervention – MICCAI 2009, Lecture Notes in Computer Science LNCS, Vol. 5762, pp. 167--174. 2009.
DOI: 10.1007/978-3-642-04271-3_21
PubMed ID: 20426109
P.T. Fletcher PT, S. Venkatasubramanian, S. Joshi.
The geometric median on Riemannian manifolds with application to robust atlas estimation, In Neuroimage, Vol. 45, No. 1, pp. S143--S152. March, 2009.
PubMed ID: 19056498
P.T. Fletcher, J. Moeller, J.M. Phillips, S. Venkatasubramanian.
Computing Hulls In Positive Definite Space, In In Proceedings of the 19th Fall Workshop on Computational Geometry, November, 2009.
C. Goodlett, P.T. Fletcher, J.H. Gilmore, G. Gerig.
Group analysis of DTI fiber tract statistics with application to neurodevelopment, In Neuroimage, Vol. 45, No. 1 (suppl 1), pp. S133--S142. 2009.
PubMed ID: 19059345
C. Goodlett, P.T. Fletcher, J.H. Gilmore, G. Gerig.
Group Analysis of DTI Fiber Tract Statistics with Application to Neurodevelopment, In NeuroImage, Vol. 45, pp. S133--S142. December, 2009.
DOI: 10.1016/j.neuroimage.2008.10.060
PubMed ID: 19059345
PubMed Central ID: PMC2727755
Diffusion tensor imaging (DTI) provides a unique source of information about the underlying tissue structure of brain white matter in vivo including both the geometry of major fiber bundles as well as quantitative information about tissue properties represented by derived tensor measures. This paper presents a method for statistical comparison of fiber bundle diffusion properties between populations of diffusion tensor images. Unbiased diffeomorphic atlas building is used to compute a normalized coordinate system for populations of diffusion images. The diffeomorphic transformations between each subject and the atlas provide spatial normalization for the comparison of tract statistics. Diffusion properties, such as fractional anisotropy (FA) and tensor norm, along fiber tracts are modeled as multivariate functions of arc length. Hypothesis testing is performed non-parametrically using permutation testing based on the Hotelling T(2) statistic. The linear discriminant embedded in the T(2) metric provides an intuitive, localized interpretation of detected differences. The proposed methodology was tested on two clinical studies of neurodevelopment. In a study of 1 and 2 year old subjects, a significant increase in FA and a correlated decrease in Frobenius norm was found in several tracts. Significant differences in neonates were found in the splenium tract between controls and subjects with isolated mild ventriculomegaly (MVM) demonstrating the potential of this method for clinical studies.
J. Hinkle, P.T. Fletcher, Brian Wang, B. Salter, S. Joshi.
4D MAP image reconstruction incorporating organ motion, In Information Processing in Medical Imaging, Lecture Notes in Computer Science LNCS, Vol. 5636, pp. 676--687. 2009.
PubMed ID: 19694303
Page 3 of 6