Research
Polynomial Regression on Riemannian Manifolds
Parametric statistical analysis of shape change is a powerful approach to extracting useful information retrospectively from clinically acquired imaging data: for instance, to quantify aging of the corpus callosum in Alzheimer's disease. Statisticians possess a set of indispensable tools for such a task, with curve-fitting regression methods among the most useful. However, nearly all of these methods suffer from the fundamental limitation that the observed data is assumed to reside in a flat Euclidean space, which is a significant obstruction when the data lie instead on a curved space such as a sphere or a space of shapes. This is almost always the case for experiments in computational anatomy, in which collected data is represented as a collection of points in a Riemannian manifold. Recently, Fletcher and Niethammer et al. have each independently initiated the theory of geodesic regression, which generalizes the notion of linear regression to Riemannian manifolds. This first-order theory is important, but its generalization to higher orders is non-trivial, and until now such generalizations have been given little consideration.We have employed a model of Riemannian polynomials, first introduced by Leite & Krakowski and based on the rolling maps of Jupp & Kent, which allows a flexible form of polynomial regression on Riemannian manifolds. This work was first presented at the FRG meeting on shape spaces in Paris June 2012 and will be published at the European Conference on Computer Vision (ECCV) 2012 in Florence Italy. I am also preparing a journal manuscript (a preprint can be found on the arXiv). |
![]() |
4D MAP Image Reconstruction from Projection Data
Phase Binning Current standard clinical practice is to use phase binned images. A set of 10 or 20 images representing the anatomy of the patient in various phases of breathing are played as a movie to give some notion of organ motion. This method assumes a cyclic breathing pattern and artifacts are introduced when that assumption breaks down. Also note that in order to properly model organ deformation, image registration techniques must be applied after reconstruction. Such techniques have no hope of overcoming problems introduced by the drastic image artifacts caused by irregular breathing in the phase binning process. In the bottom video you can see a volume rendering of the phase binned data, made using ImageVis3D. Notice the effects of noise and the lack of temporal smoothness. |
|
4D MAP Reconstruction The advantages of our method are apparent in the video. A full 3D image can be reconstructed at any given breathing amplitude, with smooth amplitude-dependence. Also note that the deformation is spatially smooth, and that no tearing or disappearing of organs seems to occur. In this example, the cine CT protocol oversampled each couch position 19 times. Due to the signal averaging which occurs in the 4D reconstruction, an SNR increase of over 4x is observed. This can ultimately lead to reduced dose to patient during image acquisition as less X-ray intensity is needed for reconstruction. Furthermore, since the 4D reconstruction process models organ motion directly, no further image registration is required for dose modelling. |
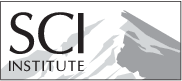

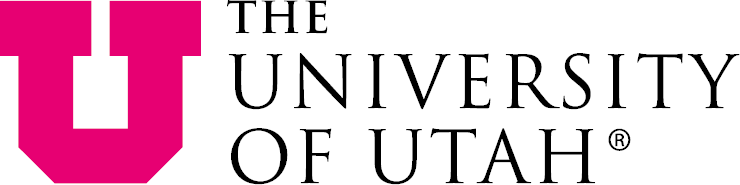